Enelaitm Agent Assist
For Calls and/or Chats of agents
Provides assistance with relevant response and Knowledge Base articles to the agent
Significantly reduces AHT by minimizing the time agents are searching & reading the KB articles
Minimizes the agents’ training requirements
Consistent CX no matter how experienced (or tired) an agent is
Can identify unknown FAQs (without articles in the KB) or sales opportunities
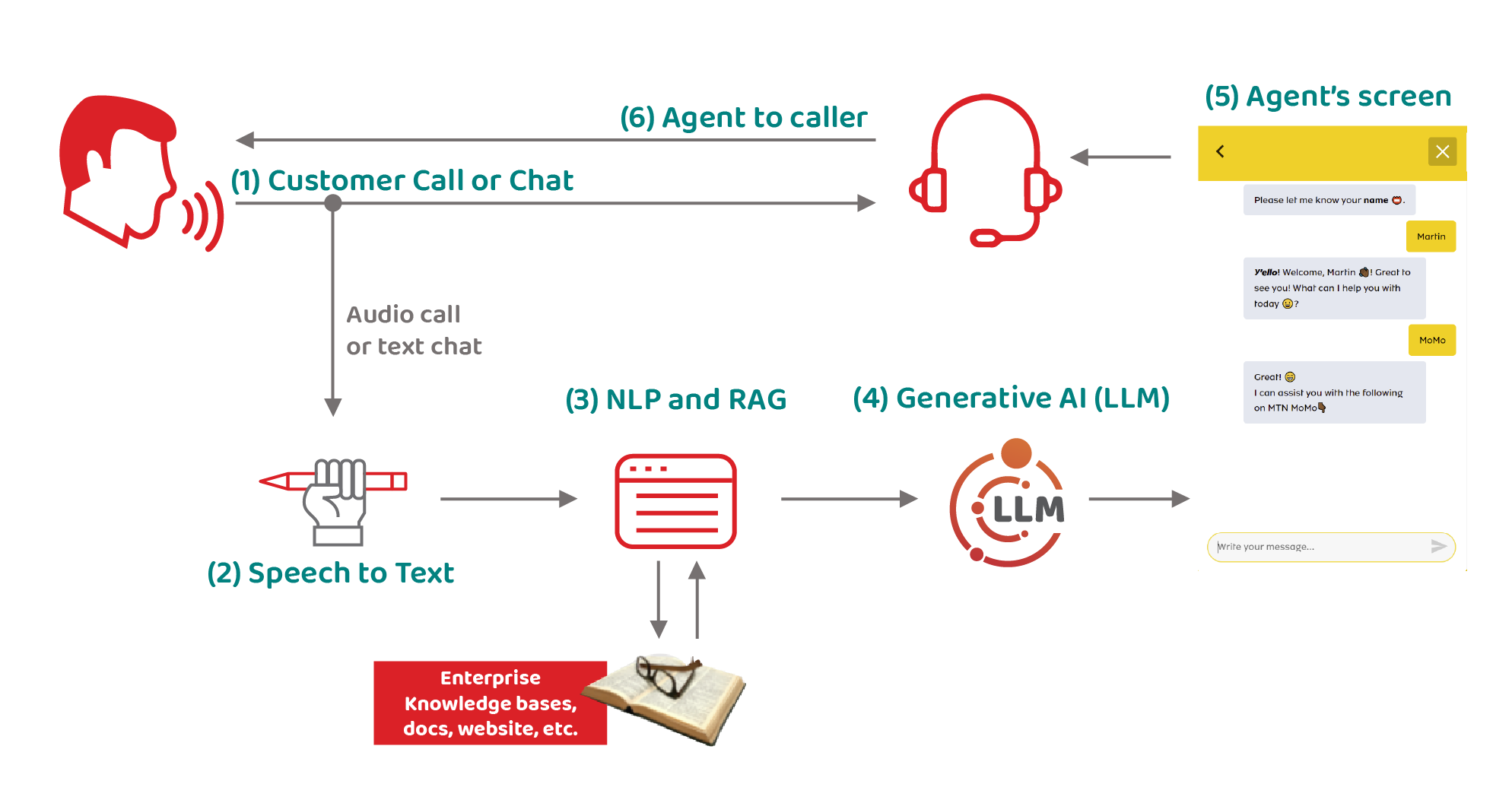
by handling up to 30% more conversations, reducing operational costs and the customers' hold time.
by at least 12% with consistent, high-quality responses and quicker training for your team.
with 15% quicker response time and fast answers to customer inquiries drawn from a central knowledge base.
Deployment modes:
(a) Entry level, as internal chatbot for Agents
Agent types 3-5 words to describe the request of the caller, and receives the proposed response and related articles in the KB. Without telephony integrations for quick and lower cost deployment.
(b) With telephony and/or LiveChat integration
- Integrated to contact center and telephony infrastructure (and/or Live Chat for chats) to capture the speech of callers (and optionally the speech of agents also) with Speech to Text for transcription of the audios.
- Responses to the agent’s desktop are produced within seconds, depending on Knowledge Base size and servers specifications.
- Optional Sentiment Analysis
- Enables advanced Speech Analytics
Driven by NLP and LLM from OpenAI, Meta (Llama2), Google or other -depending on the target language- for response generation and summarization
The RAG’s knowledge repository contains data that’s contextual to each Company and Use Case (in contrast to the data in any generalized LLM)
Data in the Knowledge Base(s) can be continually updated without incurring significant costs (and time) for LLM re-trainings
The source of the information in the vector database can be identified. And because the data sources are known, incorrect information in the KB can be corrected or deleted